Probabilistic SVM, how to get the support vectors?
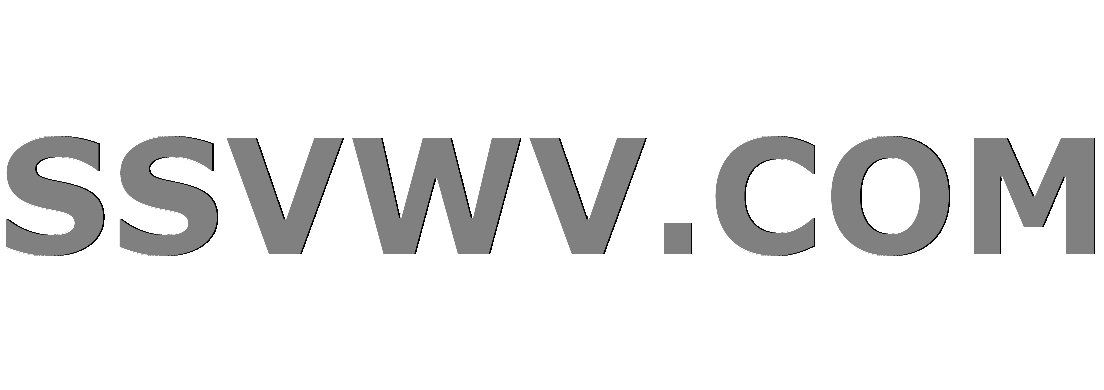
Multi tool use
Currently I'm trying to implement a probabilistic SVM, but I've run into issues, this is my code:
import numpy as np
import random
from sklearn.svm import SVC
import math
from scipy.optimize import minimize
import matplotlib.pyplot as plt
from sklearn import decomposition
from sklearn import svm
from sklearn.model_selection import GridSearchCV
from sklearn.model_selection import train_test_split
import warnings
warnings.filterwarnings("ignore")
def training_banana(name):
inputs =
file = open(name, "r")
for line in file:
vector = line.split()
coordinate =
for i in range(len(vector)):
coordinate.append(float(vector[i]))
inputs.append(coordinate)
file.close()
return np.array(inputs)
def define_inputs(name, name_targets):
inputs = training_banana(name)
targets_array = training_banana(name_targets)
N = targets_array.shape[0]
targets = np.zeros(N)
for i in range(N):
targets[i] = targets_array[i][0]
return inputs, targets, N
#training set
inputs_train, targets_train, N = define_inputs('banana_train_data_10.asc', 'banana_train_labels_10.asc') # banana_train, banana_train_label
permute = list(range(N))
random.shuffle(permute)
inputs_train = inputs_train[permute, :]
targets_train = targets_train[permute]
#test set
inputs_test, targets_test, N = define_inputs('banana_test_data_10.asc', 'banana_test_labels_10.asc') #banana_test.txt, banana_test_label
permute = list(range(N))
random.shuffle(permute)
inputs_test = inputs_test[permute, :]
targets_test = targets_test[permute]
#print(inputs_train[0])
def plotting():
param_C = [2048]
param_grid = {'C': param_C, 'kernel': ['rbf'], 'gamma': [0.5]}
clf = GridSearchCV(svm.SVC(), param_grid)
clf.fit(inputs_train, targets_train)
clf = SVC(C=clf.best_params_['C'], cache_size=200, class_weight=None, coef0=0.0,
decision_function_shape='ovr', degree=5, gamma=clf.best_params_['gamma'],
kernel=clf.best_params_['kernel'],
max_iter=-1, probability=True, random_state=None, shrinking=True,
tol=0.001, verbose=False)
clf.fit(inputs_train, targets_train)
index = clf.support_vectors_
print(len(index))
list_to_compare =
for i in range(inputs_test.shape[0]):
if clf.predict([inputs_test[i]]) == targets_test[i]:
list_to_compare.append(1)
return_value = np.sum(list_to_compare)/inputs_test.shape[0]
print(return_value)
#print(predicting_classes_pos_inputs)
#print(inputs_test)
#print(predicting_classes_pos_targets)
#print(inputs_test.shape[0])
#print(predicting_classes)
#colors = [int(i % 23) for i in xy[0]]
#for i in range(inputs_test.shape[0]):
# if clf.predict([targets_test[i]]) == 1:
# predict_pos_inputs.append(inputs_test[i])
#print(predict_pos_inputs)
#plt.scatter(inputs_test[:, 0], predictions[:, 0], s=30, cmap=plt.cm.Paired)
#plt.show()
#print(inputs_test.shape[0])
#support_vectors = get_supp_vec()
#print(support_vectors[:,0])
plotting()
My problem is that I seem to get the same number of support vectors no matter if I choose the probabilistic version or the "standard" SVM. By the probabilistic version, I mean Platt's implementation of it. As you see, I'm using:
index = clf.support_vectors_
print(len(index))
After running it on 10 datasets, I get approximately 84.7 support vectors, if I instead try using:
index2 = clf.n_support
print(len(index2))
I get only two support vectors. From what I've understood, probabilstic SVM is supposed to be more sparse than the regular, but when I use the second variant (index2), it doesn't really matter how I change gamma or C (the slack), I still get the same result, that is, complete insensitivty to the parameters, which also seems wrong.
Can someone tell me, what is the correct way of plotting the support vectors for a probabilistic SVM using the sklearn package? Thanks
python scikit-learn
New contributor
Konrad S is a new contributor to this site. Take care in asking for clarification, commenting, and answering.
Check out our Code of Conduct.
add a comment |
Currently I'm trying to implement a probabilistic SVM, but I've run into issues, this is my code:
import numpy as np
import random
from sklearn.svm import SVC
import math
from scipy.optimize import minimize
import matplotlib.pyplot as plt
from sklearn import decomposition
from sklearn import svm
from sklearn.model_selection import GridSearchCV
from sklearn.model_selection import train_test_split
import warnings
warnings.filterwarnings("ignore")
def training_banana(name):
inputs =
file = open(name, "r")
for line in file:
vector = line.split()
coordinate =
for i in range(len(vector)):
coordinate.append(float(vector[i]))
inputs.append(coordinate)
file.close()
return np.array(inputs)
def define_inputs(name, name_targets):
inputs = training_banana(name)
targets_array = training_banana(name_targets)
N = targets_array.shape[0]
targets = np.zeros(N)
for i in range(N):
targets[i] = targets_array[i][0]
return inputs, targets, N
#training set
inputs_train, targets_train, N = define_inputs('banana_train_data_10.asc', 'banana_train_labels_10.asc') # banana_train, banana_train_label
permute = list(range(N))
random.shuffle(permute)
inputs_train = inputs_train[permute, :]
targets_train = targets_train[permute]
#test set
inputs_test, targets_test, N = define_inputs('banana_test_data_10.asc', 'banana_test_labels_10.asc') #banana_test.txt, banana_test_label
permute = list(range(N))
random.shuffle(permute)
inputs_test = inputs_test[permute, :]
targets_test = targets_test[permute]
#print(inputs_train[0])
def plotting():
param_C = [2048]
param_grid = {'C': param_C, 'kernel': ['rbf'], 'gamma': [0.5]}
clf = GridSearchCV(svm.SVC(), param_grid)
clf.fit(inputs_train, targets_train)
clf = SVC(C=clf.best_params_['C'], cache_size=200, class_weight=None, coef0=0.0,
decision_function_shape='ovr', degree=5, gamma=clf.best_params_['gamma'],
kernel=clf.best_params_['kernel'],
max_iter=-1, probability=True, random_state=None, shrinking=True,
tol=0.001, verbose=False)
clf.fit(inputs_train, targets_train)
index = clf.support_vectors_
print(len(index))
list_to_compare =
for i in range(inputs_test.shape[0]):
if clf.predict([inputs_test[i]]) == targets_test[i]:
list_to_compare.append(1)
return_value = np.sum(list_to_compare)/inputs_test.shape[0]
print(return_value)
#print(predicting_classes_pos_inputs)
#print(inputs_test)
#print(predicting_classes_pos_targets)
#print(inputs_test.shape[0])
#print(predicting_classes)
#colors = [int(i % 23) for i in xy[0]]
#for i in range(inputs_test.shape[0]):
# if clf.predict([targets_test[i]]) == 1:
# predict_pos_inputs.append(inputs_test[i])
#print(predict_pos_inputs)
#plt.scatter(inputs_test[:, 0], predictions[:, 0], s=30, cmap=plt.cm.Paired)
#plt.show()
#print(inputs_test.shape[0])
#support_vectors = get_supp_vec()
#print(support_vectors[:,0])
plotting()
My problem is that I seem to get the same number of support vectors no matter if I choose the probabilistic version or the "standard" SVM. By the probabilistic version, I mean Platt's implementation of it. As you see, I'm using:
index = clf.support_vectors_
print(len(index))
After running it on 10 datasets, I get approximately 84.7 support vectors, if I instead try using:
index2 = clf.n_support
print(len(index2))
I get only two support vectors. From what I've understood, probabilstic SVM is supposed to be more sparse than the regular, but when I use the second variant (index2), it doesn't really matter how I change gamma or C (the slack), I still get the same result, that is, complete insensitivty to the parameters, which also seems wrong.
Can someone tell me, what is the correct way of plotting the support vectors for a probabilistic SVM using the sklearn package? Thanks
python scikit-learn
New contributor
Konrad S is a new contributor to this site. Take care in asking for clarification, commenting, and answering.
Check out our Code of Conduct.
I suppose I've done wrong and I should've went for a SMO if I really wanted a probabilistic output?
– Konrad S
yesterday
SVC(probability=True)
does not change the fitting of SVC in any way. It just calculates the probabilities for the samples predicted after the fitting from the learned model. So that will not change the number of support vectors.
– Vivek Kumar
yesterday
add a comment |
Currently I'm trying to implement a probabilistic SVM, but I've run into issues, this is my code:
import numpy as np
import random
from sklearn.svm import SVC
import math
from scipy.optimize import minimize
import matplotlib.pyplot as plt
from sklearn import decomposition
from sklearn import svm
from sklearn.model_selection import GridSearchCV
from sklearn.model_selection import train_test_split
import warnings
warnings.filterwarnings("ignore")
def training_banana(name):
inputs =
file = open(name, "r")
for line in file:
vector = line.split()
coordinate =
for i in range(len(vector)):
coordinate.append(float(vector[i]))
inputs.append(coordinate)
file.close()
return np.array(inputs)
def define_inputs(name, name_targets):
inputs = training_banana(name)
targets_array = training_banana(name_targets)
N = targets_array.shape[0]
targets = np.zeros(N)
for i in range(N):
targets[i] = targets_array[i][0]
return inputs, targets, N
#training set
inputs_train, targets_train, N = define_inputs('banana_train_data_10.asc', 'banana_train_labels_10.asc') # banana_train, banana_train_label
permute = list(range(N))
random.shuffle(permute)
inputs_train = inputs_train[permute, :]
targets_train = targets_train[permute]
#test set
inputs_test, targets_test, N = define_inputs('banana_test_data_10.asc', 'banana_test_labels_10.asc') #banana_test.txt, banana_test_label
permute = list(range(N))
random.shuffle(permute)
inputs_test = inputs_test[permute, :]
targets_test = targets_test[permute]
#print(inputs_train[0])
def plotting():
param_C = [2048]
param_grid = {'C': param_C, 'kernel': ['rbf'], 'gamma': [0.5]}
clf = GridSearchCV(svm.SVC(), param_grid)
clf.fit(inputs_train, targets_train)
clf = SVC(C=clf.best_params_['C'], cache_size=200, class_weight=None, coef0=0.0,
decision_function_shape='ovr', degree=5, gamma=clf.best_params_['gamma'],
kernel=clf.best_params_['kernel'],
max_iter=-1, probability=True, random_state=None, shrinking=True,
tol=0.001, verbose=False)
clf.fit(inputs_train, targets_train)
index = clf.support_vectors_
print(len(index))
list_to_compare =
for i in range(inputs_test.shape[0]):
if clf.predict([inputs_test[i]]) == targets_test[i]:
list_to_compare.append(1)
return_value = np.sum(list_to_compare)/inputs_test.shape[0]
print(return_value)
#print(predicting_classes_pos_inputs)
#print(inputs_test)
#print(predicting_classes_pos_targets)
#print(inputs_test.shape[0])
#print(predicting_classes)
#colors = [int(i % 23) for i in xy[0]]
#for i in range(inputs_test.shape[0]):
# if clf.predict([targets_test[i]]) == 1:
# predict_pos_inputs.append(inputs_test[i])
#print(predict_pos_inputs)
#plt.scatter(inputs_test[:, 0], predictions[:, 0], s=30, cmap=plt.cm.Paired)
#plt.show()
#print(inputs_test.shape[0])
#support_vectors = get_supp_vec()
#print(support_vectors[:,0])
plotting()
My problem is that I seem to get the same number of support vectors no matter if I choose the probabilistic version or the "standard" SVM. By the probabilistic version, I mean Platt's implementation of it. As you see, I'm using:
index = clf.support_vectors_
print(len(index))
After running it on 10 datasets, I get approximately 84.7 support vectors, if I instead try using:
index2 = clf.n_support
print(len(index2))
I get only two support vectors. From what I've understood, probabilstic SVM is supposed to be more sparse than the regular, but when I use the second variant (index2), it doesn't really matter how I change gamma or C (the slack), I still get the same result, that is, complete insensitivty to the parameters, which also seems wrong.
Can someone tell me, what is the correct way of plotting the support vectors for a probabilistic SVM using the sklearn package? Thanks
python scikit-learn
New contributor
Konrad S is a new contributor to this site. Take care in asking for clarification, commenting, and answering.
Check out our Code of Conduct.
Currently I'm trying to implement a probabilistic SVM, but I've run into issues, this is my code:
import numpy as np
import random
from sklearn.svm import SVC
import math
from scipy.optimize import minimize
import matplotlib.pyplot as plt
from sklearn import decomposition
from sklearn import svm
from sklearn.model_selection import GridSearchCV
from sklearn.model_selection import train_test_split
import warnings
warnings.filterwarnings("ignore")
def training_banana(name):
inputs =
file = open(name, "r")
for line in file:
vector = line.split()
coordinate =
for i in range(len(vector)):
coordinate.append(float(vector[i]))
inputs.append(coordinate)
file.close()
return np.array(inputs)
def define_inputs(name, name_targets):
inputs = training_banana(name)
targets_array = training_banana(name_targets)
N = targets_array.shape[0]
targets = np.zeros(N)
for i in range(N):
targets[i] = targets_array[i][0]
return inputs, targets, N
#training set
inputs_train, targets_train, N = define_inputs('banana_train_data_10.asc', 'banana_train_labels_10.asc') # banana_train, banana_train_label
permute = list(range(N))
random.shuffle(permute)
inputs_train = inputs_train[permute, :]
targets_train = targets_train[permute]
#test set
inputs_test, targets_test, N = define_inputs('banana_test_data_10.asc', 'banana_test_labels_10.asc') #banana_test.txt, banana_test_label
permute = list(range(N))
random.shuffle(permute)
inputs_test = inputs_test[permute, :]
targets_test = targets_test[permute]
#print(inputs_train[0])
def plotting():
param_C = [2048]
param_grid = {'C': param_C, 'kernel': ['rbf'], 'gamma': [0.5]}
clf = GridSearchCV(svm.SVC(), param_grid)
clf.fit(inputs_train, targets_train)
clf = SVC(C=clf.best_params_['C'], cache_size=200, class_weight=None, coef0=0.0,
decision_function_shape='ovr', degree=5, gamma=clf.best_params_['gamma'],
kernel=clf.best_params_['kernel'],
max_iter=-1, probability=True, random_state=None, shrinking=True,
tol=0.001, verbose=False)
clf.fit(inputs_train, targets_train)
index = clf.support_vectors_
print(len(index))
list_to_compare =
for i in range(inputs_test.shape[0]):
if clf.predict([inputs_test[i]]) == targets_test[i]:
list_to_compare.append(1)
return_value = np.sum(list_to_compare)/inputs_test.shape[0]
print(return_value)
#print(predicting_classes_pos_inputs)
#print(inputs_test)
#print(predicting_classes_pos_targets)
#print(inputs_test.shape[0])
#print(predicting_classes)
#colors = [int(i % 23) for i in xy[0]]
#for i in range(inputs_test.shape[0]):
# if clf.predict([targets_test[i]]) == 1:
# predict_pos_inputs.append(inputs_test[i])
#print(predict_pos_inputs)
#plt.scatter(inputs_test[:, 0], predictions[:, 0], s=30, cmap=plt.cm.Paired)
#plt.show()
#print(inputs_test.shape[0])
#support_vectors = get_supp_vec()
#print(support_vectors[:,0])
plotting()
My problem is that I seem to get the same number of support vectors no matter if I choose the probabilistic version or the "standard" SVM. By the probabilistic version, I mean Platt's implementation of it. As you see, I'm using:
index = clf.support_vectors_
print(len(index))
After running it on 10 datasets, I get approximately 84.7 support vectors, if I instead try using:
index2 = clf.n_support
print(len(index2))
I get only two support vectors. From what I've understood, probabilstic SVM is supposed to be more sparse than the regular, but when I use the second variant (index2), it doesn't really matter how I change gamma or C (the slack), I still get the same result, that is, complete insensitivty to the parameters, which also seems wrong.
Can someone tell me, what is the correct way of plotting the support vectors for a probabilistic SVM using the sklearn package? Thanks
python scikit-learn
python scikit-learn
New contributor
Konrad S is a new contributor to this site. Take care in asking for clarification, commenting, and answering.
Check out our Code of Conduct.
New contributor
Konrad S is a new contributor to this site. Take care in asking for clarification, commenting, and answering.
Check out our Code of Conduct.
edited yesterday


Vinay Bharadhwaj
11111
11111
New contributor
Konrad S is a new contributor to this site. Take care in asking for clarification, commenting, and answering.
Check out our Code of Conduct.
asked yesterday
Konrad SKonrad S
1
1
New contributor
Konrad S is a new contributor to this site. Take care in asking for clarification, commenting, and answering.
Check out our Code of Conduct.
New contributor
Konrad S is a new contributor to this site. Take care in asking for clarification, commenting, and answering.
Check out our Code of Conduct.
Konrad S is a new contributor to this site. Take care in asking for clarification, commenting, and answering.
Check out our Code of Conduct.
I suppose I've done wrong and I should've went for a SMO if I really wanted a probabilistic output?
– Konrad S
yesterday
SVC(probability=True)
does not change the fitting of SVC in any way. It just calculates the probabilities for the samples predicted after the fitting from the learned model. So that will not change the number of support vectors.
– Vivek Kumar
yesterday
add a comment |
I suppose I've done wrong and I should've went for a SMO if I really wanted a probabilistic output?
– Konrad S
yesterday
SVC(probability=True)
does not change the fitting of SVC in any way. It just calculates the probabilities for the samples predicted after the fitting from the learned model. So that will not change the number of support vectors.
– Vivek Kumar
yesterday
I suppose I've done wrong and I should've went for a SMO if I really wanted a probabilistic output?
– Konrad S
yesterday
I suppose I've done wrong and I should've went for a SMO if I really wanted a probabilistic output?
– Konrad S
yesterday
SVC(probability=True)
does not change the fitting of SVC in any way. It just calculates the probabilities for the samples predicted after the fitting from the learned model. So that will not change the number of support vectors.– Vivek Kumar
yesterday
SVC(probability=True)
does not change the fitting of SVC in any way. It just calculates the probabilities for the samples predicted after the fitting from the learned model. So that will not change the number of support vectors.– Vivek Kumar
yesterday
add a comment |
0
active
oldest
votes
Your Answer
StackExchange.ifUsing("editor", function () {
StackExchange.using("externalEditor", function () {
StackExchange.using("snippets", function () {
StackExchange.snippets.init();
});
});
}, "code-snippets");
StackExchange.ready(function() {
var channelOptions = {
tags: "".split(" "),
id: "1"
};
initTagRenderer("".split(" "), "".split(" "), channelOptions);
StackExchange.using("externalEditor", function() {
// Have to fire editor after snippets, if snippets enabled
if (StackExchange.settings.snippets.snippetsEnabled) {
StackExchange.using("snippets", function() {
createEditor();
});
}
else {
createEditor();
}
});
function createEditor() {
StackExchange.prepareEditor({
heartbeatType: 'answer',
autoActivateHeartbeat: false,
convertImagesToLinks: true,
noModals: true,
showLowRepImageUploadWarning: true,
reputationToPostImages: 10,
bindNavPrevention: true,
postfix: "",
imageUploader: {
brandingHtml: "Powered by u003ca class="icon-imgur-white" href="https://imgur.com/"u003eu003c/au003e",
contentPolicyHtml: "User contributions licensed under u003ca href="https://creativecommons.org/licenses/by-sa/3.0/"u003ecc by-sa 3.0 with attribution requiredu003c/au003e u003ca href="https://stackoverflow.com/legal/content-policy"u003e(content policy)u003c/au003e",
allowUrls: true
},
onDemand: true,
discardSelector: ".discard-answer"
,immediatelyShowMarkdownHelp:true
});
}
});
Konrad S is a new contributor. Be nice, and check out our Code of Conduct.
Sign up or log in
StackExchange.ready(function () {
StackExchange.helpers.onClickDraftSave('#login-link');
});
Sign up using Google
Sign up using Facebook
Sign up using Email and Password
Post as a guest
Required, but never shown
StackExchange.ready(
function () {
StackExchange.openid.initPostLogin('.new-post-login', 'https%3a%2f%2fstackoverflow.com%2fquestions%2f54251895%2fprobabilistic-svm-how-to-get-the-support-vectors%23new-answer', 'question_page');
}
);
Post as a guest
Required, but never shown
0
active
oldest
votes
0
active
oldest
votes
active
oldest
votes
active
oldest
votes
Konrad S is a new contributor. Be nice, and check out our Code of Conduct.
Konrad S is a new contributor. Be nice, and check out our Code of Conduct.
Konrad S is a new contributor. Be nice, and check out our Code of Conduct.
Konrad S is a new contributor. Be nice, and check out our Code of Conduct.
Thanks for contributing an answer to Stack Overflow!
- Please be sure to answer the question. Provide details and share your research!
But avoid …
- Asking for help, clarification, or responding to other answers.
- Making statements based on opinion; back them up with references or personal experience.
To learn more, see our tips on writing great answers.
Sign up or log in
StackExchange.ready(function () {
StackExchange.helpers.onClickDraftSave('#login-link');
});
Sign up using Google
Sign up using Facebook
Sign up using Email and Password
Post as a guest
Required, but never shown
StackExchange.ready(
function () {
StackExchange.openid.initPostLogin('.new-post-login', 'https%3a%2f%2fstackoverflow.com%2fquestions%2f54251895%2fprobabilistic-svm-how-to-get-the-support-vectors%23new-answer', 'question_page');
}
);
Post as a guest
Required, but never shown
Sign up or log in
StackExchange.ready(function () {
StackExchange.helpers.onClickDraftSave('#login-link');
});
Sign up using Google
Sign up using Facebook
Sign up using Email and Password
Post as a guest
Required, but never shown
Sign up or log in
StackExchange.ready(function () {
StackExchange.helpers.onClickDraftSave('#login-link');
});
Sign up using Google
Sign up using Facebook
Sign up using Email and Password
Post as a guest
Required, but never shown
Sign up or log in
StackExchange.ready(function () {
StackExchange.helpers.onClickDraftSave('#login-link');
});
Sign up using Google
Sign up using Facebook
Sign up using Email and Password
Sign up using Google
Sign up using Facebook
Sign up using Email and Password
Post as a guest
Required, but never shown
Required, but never shown
Required, but never shown
Required, but never shown
Required, but never shown
Required, but never shown
Required, but never shown
Required, but never shown
Required, but never shown
H J bGkUvAJpnyENh04D kw,ih95UFGdCE4ocQvsbmkOtk,lIV0
I suppose I've done wrong and I should've went for a SMO if I really wanted a probabilistic output?
– Konrad S
yesterday
SVC(probability=True)
does not change the fitting of SVC in any way. It just calculates the probabilities for the samples predicted after the fitting from the learned model. So that will not change the number of support vectors.– Vivek Kumar
yesterday