Find Most Important Input from a Neural Network
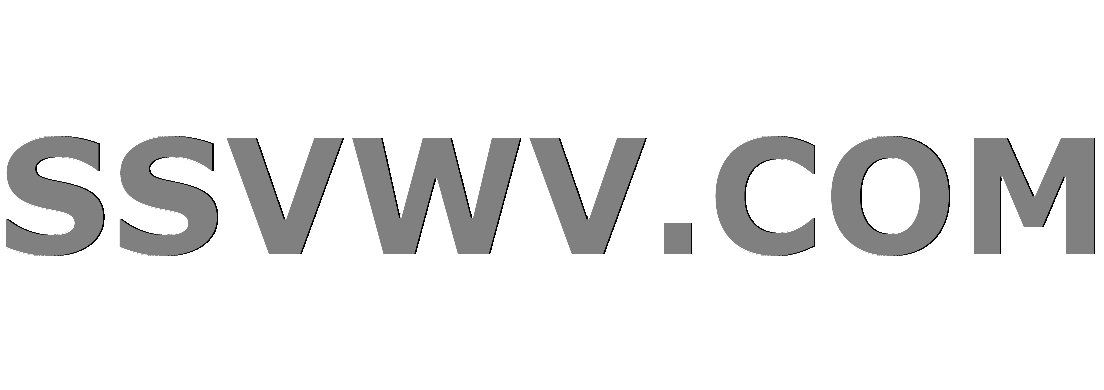
Multi tool use
I trained a neural network with 37 Inputs. It has around 85% accuracy. Is it possible for me to find out which Input has the most effect. I tried this code but I cannot figure out how to find most important Input
weights = model.layers[0].get_weights()[0]
biases = model.layers[0].get_weights()[1]
python-3.x tensorflow keras
add a comment |
I trained a neural network with 37 Inputs. It has around 85% accuracy. Is it possible for me to find out which Input has the most effect. I tried this code but I cannot figure out how to find most important Input
weights = model.layers[0].get_weights()[0]
biases = model.layers[0].get_weights()[1]
python-3.x tensorflow keras
add a comment |
I trained a neural network with 37 Inputs. It has around 85% accuracy. Is it possible for me to find out which Input has the most effect. I tried this code but I cannot figure out how to find most important Input
weights = model.layers[0].get_weights()[0]
biases = model.layers[0].get_weights()[1]
python-3.x tensorflow keras
I trained a neural network with 37 Inputs. It has around 85% accuracy. Is it possible for me to find out which Input has the most effect. I tried this code but I cannot figure out how to find most important Input
weights = model.layers[0].get_weights()[0]
biases = model.layers[0].get_weights()[1]
python-3.x tensorflow keras
python-3.x tensorflow keras
asked Jan 20 at 3:25
APP BirdAPP Bird
6861025
6861025
add a comment |
add a comment |
1 Answer
1
active
oldest
votes
One possible solution is to wrap your model with keras.wrappers.scikit_learn
and then use Recursive Feature elimination in scikit-learn:
def create_model():
# create model
model = Sequential()
model.add(Dense(512, activation='relu'))
model.add(Dense(512, activation='relu'))
model.add(Dense(10, activation='softmax'))
# Compile model
model.compile(loss='categorical_crossentropy', optimizer='adam', metrics=['accuracy'])
return model
model = KerasClassifier(build_fn=create_model, epochs=100, batch_size=128, verbose=0)
rfe = RFE(estimator=model, n_features_to_select=1, step=1)
rfe.fit(X, y)
ranking = rfe.ranking_.reshape(digits.images[0].shape)
# Plot pixel ranking
plt.matshow(ranking, cmap=plt.cm.Blues)
plt.colorbar()
plt.title("Ranking of pixels with RFE")
plt.show()
If you need to visualize weights see here.
add a comment |
Your Answer
StackExchange.ifUsing("editor", function () {
StackExchange.using("externalEditor", function () {
StackExchange.using("snippets", function () {
StackExchange.snippets.init();
});
});
}, "code-snippets");
StackExchange.ready(function() {
var channelOptions = {
tags: "".split(" "),
id: "1"
};
initTagRenderer("".split(" "), "".split(" "), channelOptions);
StackExchange.using("externalEditor", function() {
// Have to fire editor after snippets, if snippets enabled
if (StackExchange.settings.snippets.snippetsEnabled) {
StackExchange.using("snippets", function() {
createEditor();
});
}
else {
createEditor();
}
});
function createEditor() {
StackExchange.prepareEditor({
heartbeatType: 'answer',
autoActivateHeartbeat: false,
convertImagesToLinks: true,
noModals: true,
showLowRepImageUploadWarning: true,
reputationToPostImages: 10,
bindNavPrevention: true,
postfix: "",
imageUploader: {
brandingHtml: "Powered by u003ca class="icon-imgur-white" href="https://imgur.com/"u003eu003c/au003e",
contentPolicyHtml: "User contributions licensed under u003ca href="https://creativecommons.org/licenses/by-sa/3.0/"u003ecc by-sa 3.0 with attribution requiredu003c/au003e u003ca href="https://stackoverflow.com/legal/content-policy"u003e(content policy)u003c/au003e",
allowUrls: true
},
onDemand: true,
discardSelector: ".discard-answer"
,immediatelyShowMarkdownHelp:true
});
}
});
Sign up or log in
StackExchange.ready(function () {
StackExchange.helpers.onClickDraftSave('#login-link');
});
Sign up using Google
Sign up using Facebook
Sign up using Email and Password
Post as a guest
Required, but never shown
StackExchange.ready(
function () {
StackExchange.openid.initPostLogin('.new-post-login', 'https%3a%2f%2fstackoverflow.com%2fquestions%2f54273338%2ffind-most-important-input-from-a-neural-network%23new-answer', 'question_page');
}
);
Post as a guest
Required, but never shown
1 Answer
1
active
oldest
votes
1 Answer
1
active
oldest
votes
active
oldest
votes
active
oldest
votes
One possible solution is to wrap your model with keras.wrappers.scikit_learn
and then use Recursive Feature elimination in scikit-learn:
def create_model():
# create model
model = Sequential()
model.add(Dense(512, activation='relu'))
model.add(Dense(512, activation='relu'))
model.add(Dense(10, activation='softmax'))
# Compile model
model.compile(loss='categorical_crossentropy', optimizer='adam', metrics=['accuracy'])
return model
model = KerasClassifier(build_fn=create_model, epochs=100, batch_size=128, verbose=0)
rfe = RFE(estimator=model, n_features_to_select=1, step=1)
rfe.fit(X, y)
ranking = rfe.ranking_.reshape(digits.images[0].shape)
# Plot pixel ranking
plt.matshow(ranking, cmap=plt.cm.Blues)
plt.colorbar()
plt.title("Ranking of pixels with RFE")
plt.show()
If you need to visualize weights see here.
add a comment |
One possible solution is to wrap your model with keras.wrappers.scikit_learn
and then use Recursive Feature elimination in scikit-learn:
def create_model():
# create model
model = Sequential()
model.add(Dense(512, activation='relu'))
model.add(Dense(512, activation='relu'))
model.add(Dense(10, activation='softmax'))
# Compile model
model.compile(loss='categorical_crossentropy', optimizer='adam', metrics=['accuracy'])
return model
model = KerasClassifier(build_fn=create_model, epochs=100, batch_size=128, verbose=0)
rfe = RFE(estimator=model, n_features_to_select=1, step=1)
rfe.fit(X, y)
ranking = rfe.ranking_.reshape(digits.images[0].shape)
# Plot pixel ranking
plt.matshow(ranking, cmap=plt.cm.Blues)
plt.colorbar()
plt.title("Ranking of pixels with RFE")
plt.show()
If you need to visualize weights see here.
add a comment |
One possible solution is to wrap your model with keras.wrappers.scikit_learn
and then use Recursive Feature elimination in scikit-learn:
def create_model():
# create model
model = Sequential()
model.add(Dense(512, activation='relu'))
model.add(Dense(512, activation='relu'))
model.add(Dense(10, activation='softmax'))
# Compile model
model.compile(loss='categorical_crossentropy', optimizer='adam', metrics=['accuracy'])
return model
model = KerasClassifier(build_fn=create_model, epochs=100, batch_size=128, verbose=0)
rfe = RFE(estimator=model, n_features_to_select=1, step=1)
rfe.fit(X, y)
ranking = rfe.ranking_.reshape(digits.images[0].shape)
# Plot pixel ranking
plt.matshow(ranking, cmap=plt.cm.Blues)
plt.colorbar()
plt.title("Ranking of pixels with RFE")
plt.show()
If you need to visualize weights see here.
One possible solution is to wrap your model with keras.wrappers.scikit_learn
and then use Recursive Feature elimination in scikit-learn:
def create_model():
# create model
model = Sequential()
model.add(Dense(512, activation='relu'))
model.add(Dense(512, activation='relu'))
model.add(Dense(10, activation='softmax'))
# Compile model
model.compile(loss='categorical_crossentropy', optimizer='adam', metrics=['accuracy'])
return model
model = KerasClassifier(build_fn=create_model, epochs=100, batch_size=128, verbose=0)
rfe = RFE(estimator=model, n_features_to_select=1, step=1)
rfe.fit(X, y)
ranking = rfe.ranking_.reshape(digits.images[0].shape)
# Plot pixel ranking
plt.matshow(ranking, cmap=plt.cm.Blues)
plt.colorbar()
plt.title("Ranking of pixels with RFE")
plt.show()
If you need to visualize weights see here.
answered Jan 20 at 8:00


AmirAmir
7,71264173
7,71264173
add a comment |
add a comment |
Thanks for contributing an answer to Stack Overflow!
- Please be sure to answer the question. Provide details and share your research!
But avoid …
- Asking for help, clarification, or responding to other answers.
- Making statements based on opinion; back them up with references or personal experience.
To learn more, see our tips on writing great answers.
Sign up or log in
StackExchange.ready(function () {
StackExchange.helpers.onClickDraftSave('#login-link');
});
Sign up using Google
Sign up using Facebook
Sign up using Email and Password
Post as a guest
Required, but never shown
StackExchange.ready(
function () {
StackExchange.openid.initPostLogin('.new-post-login', 'https%3a%2f%2fstackoverflow.com%2fquestions%2f54273338%2ffind-most-important-input-from-a-neural-network%23new-answer', 'question_page');
}
);
Post as a guest
Required, but never shown
Sign up or log in
StackExchange.ready(function () {
StackExchange.helpers.onClickDraftSave('#login-link');
});
Sign up using Google
Sign up using Facebook
Sign up using Email and Password
Post as a guest
Required, but never shown
Sign up or log in
StackExchange.ready(function () {
StackExchange.helpers.onClickDraftSave('#login-link');
});
Sign up using Google
Sign up using Facebook
Sign up using Email and Password
Post as a guest
Required, but never shown
Sign up or log in
StackExchange.ready(function () {
StackExchange.helpers.onClickDraftSave('#login-link');
});
Sign up using Google
Sign up using Facebook
Sign up using Email and Password
Sign up using Google
Sign up using Facebook
Sign up using Email and Password
Post as a guest
Required, but never shown
Required, but never shown
Required, but never shown
Required, but never shown
Required, but never shown
Required, but never shown
Required, but never shown
Required, but never shown
Required, but never shown
ZwFoVQNkxL