Rolling multidimensional function in pandas
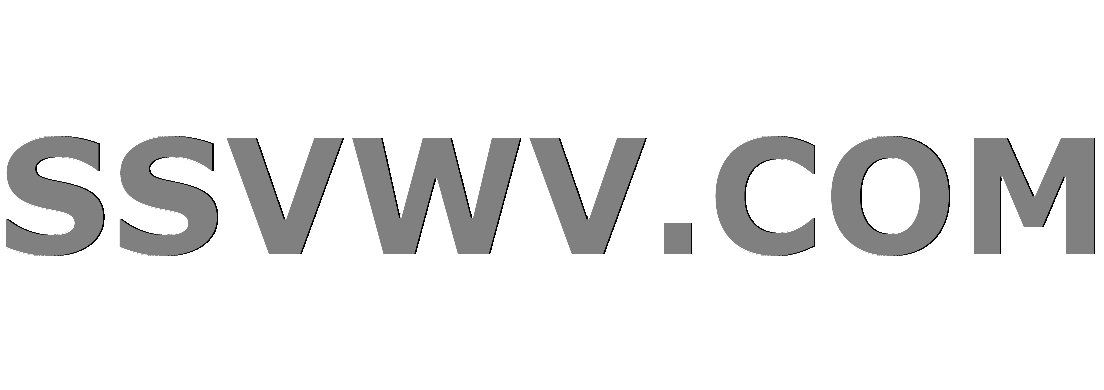
Multi tool use
Let's say, I have the following code.
import numpy as np
import pandas as pd
x = pd.DataFrame(np.random.randn(100, 3)).rolling(window=10, center=True).cov()
For each index, I have a 3x3 matrix. I would like to calculate eigenvalues and then some function of those eigenvalues. Or, perhaps, I might want to compute some function of eigenvalues and eigenvectors. The point is that if I take x.loc[0] then I have no problem to compute anything from that matrix. How do I do it in a rolling fashion for all matrices?
Thanks!
pandas rolling
add a comment |
Let's say, I have the following code.
import numpy as np
import pandas as pd
x = pd.DataFrame(np.random.randn(100, 3)).rolling(window=10, center=True).cov()
For each index, I have a 3x3 matrix. I would like to calculate eigenvalues and then some function of those eigenvalues. Or, perhaps, I might want to compute some function of eigenvalues and eigenvectors. The point is that if I take x.loc[0] then I have no problem to compute anything from that matrix. How do I do it in a rolling fashion for all matrices?
Thanks!
pandas rolling
add a comment |
Let's say, I have the following code.
import numpy as np
import pandas as pd
x = pd.DataFrame(np.random.randn(100, 3)).rolling(window=10, center=True).cov()
For each index, I have a 3x3 matrix. I would like to calculate eigenvalues and then some function of those eigenvalues. Or, perhaps, I might want to compute some function of eigenvalues and eigenvectors. The point is that if I take x.loc[0] then I have no problem to compute anything from that matrix. How do I do it in a rolling fashion for all matrices?
Thanks!
pandas rolling
Let's say, I have the following code.
import numpy as np
import pandas as pd
x = pd.DataFrame(np.random.randn(100, 3)).rolling(window=10, center=True).cov()
For each index, I have a 3x3 matrix. I would like to calculate eigenvalues and then some function of those eigenvalues. Or, perhaps, I might want to compute some function of eigenvalues and eigenvectors. The point is that if I take x.loc[0] then I have no problem to compute anything from that matrix. How do I do it in a rolling fashion for all matrices?
Thanks!
pandas rolling
pandas rolling
asked Jan 20 at 3:25
freevillagefreevillage
1
1
add a comment |
add a comment |
1 Answer
1
active
oldest
votes
You can use the analogous eigenvector/eigenvalue methods in spicy.sparse.linalg.
import numpy as np
import pandas as pd
from scipy import linalg as LA
x = pd.DataFrame(np.random.randn(100, 3)).rolling(window=10, center=True).cov()
for i in range(len(x)):
try:
e_vals,e_vec = LA.eig(x.loc[i])
print(e_vals,e_vec)
except:
continue
If there are no NaN values present then you need not use the try and except instead go for only for loop.
What I don't understand is why I can calculate a covariance matrix for each "window x 3" window but I cannot calculate a more general function. Of course, I can do another loop after that but it seems wasteful. Why go over the matrix twice?
– freevillage
Jan 21 at 0:46
add a comment |
Your Answer
StackExchange.ifUsing("editor", function () {
StackExchange.using("externalEditor", function () {
StackExchange.using("snippets", function () {
StackExchange.snippets.init();
});
});
}, "code-snippets");
StackExchange.ready(function() {
var channelOptions = {
tags: "".split(" "),
id: "1"
};
initTagRenderer("".split(" "), "".split(" "), channelOptions);
StackExchange.using("externalEditor", function() {
// Have to fire editor after snippets, if snippets enabled
if (StackExchange.settings.snippets.snippetsEnabled) {
StackExchange.using("snippets", function() {
createEditor();
});
}
else {
createEditor();
}
});
function createEditor() {
StackExchange.prepareEditor({
heartbeatType: 'answer',
autoActivateHeartbeat: false,
convertImagesToLinks: true,
noModals: true,
showLowRepImageUploadWarning: true,
reputationToPostImages: 10,
bindNavPrevention: true,
postfix: "",
imageUploader: {
brandingHtml: "Powered by u003ca class="icon-imgur-white" href="https://imgur.com/"u003eu003c/au003e",
contentPolicyHtml: "User contributions licensed under u003ca href="https://creativecommons.org/licenses/by-sa/3.0/"u003ecc by-sa 3.0 with attribution requiredu003c/au003e u003ca href="https://stackoverflow.com/legal/content-policy"u003e(content policy)u003c/au003e",
allowUrls: true
},
onDemand: true,
discardSelector: ".discard-answer"
,immediatelyShowMarkdownHelp:true
});
}
});
Sign up or log in
StackExchange.ready(function () {
StackExchange.helpers.onClickDraftSave('#login-link');
});
Sign up using Google
Sign up using Facebook
Sign up using Email and Password
Post as a guest
Required, but never shown
StackExchange.ready(
function () {
StackExchange.openid.initPostLogin('.new-post-login', 'https%3a%2f%2fstackoverflow.com%2fquestions%2f54273340%2frolling-multidimensional-function-in-pandas%23new-answer', 'question_page');
}
);
Post as a guest
Required, but never shown
1 Answer
1
active
oldest
votes
1 Answer
1
active
oldest
votes
active
oldest
votes
active
oldest
votes
You can use the analogous eigenvector/eigenvalue methods in spicy.sparse.linalg.
import numpy as np
import pandas as pd
from scipy import linalg as LA
x = pd.DataFrame(np.random.randn(100, 3)).rolling(window=10, center=True).cov()
for i in range(len(x)):
try:
e_vals,e_vec = LA.eig(x.loc[i])
print(e_vals,e_vec)
except:
continue
If there are no NaN values present then you need not use the try and except instead go for only for loop.
What I don't understand is why I can calculate a covariance matrix for each "window x 3" window but I cannot calculate a more general function. Of course, I can do another loop after that but it seems wasteful. Why go over the matrix twice?
– freevillage
Jan 21 at 0:46
add a comment |
You can use the analogous eigenvector/eigenvalue methods in spicy.sparse.linalg.
import numpy as np
import pandas as pd
from scipy import linalg as LA
x = pd.DataFrame(np.random.randn(100, 3)).rolling(window=10, center=True).cov()
for i in range(len(x)):
try:
e_vals,e_vec = LA.eig(x.loc[i])
print(e_vals,e_vec)
except:
continue
If there are no NaN values present then you need not use the try and except instead go for only for loop.
What I don't understand is why I can calculate a covariance matrix for each "window x 3" window but I cannot calculate a more general function. Of course, I can do another loop after that but it seems wasteful. Why go over the matrix twice?
– freevillage
Jan 21 at 0:46
add a comment |
You can use the analogous eigenvector/eigenvalue methods in spicy.sparse.linalg.
import numpy as np
import pandas as pd
from scipy import linalg as LA
x = pd.DataFrame(np.random.randn(100, 3)).rolling(window=10, center=True).cov()
for i in range(len(x)):
try:
e_vals,e_vec = LA.eig(x.loc[i])
print(e_vals,e_vec)
except:
continue
If there are no NaN values present then you need not use the try and except instead go for only for loop.
You can use the analogous eigenvector/eigenvalue methods in spicy.sparse.linalg.
import numpy as np
import pandas as pd
from scipy import linalg as LA
x = pd.DataFrame(np.random.randn(100, 3)).rolling(window=10, center=True).cov()
for i in range(len(x)):
try:
e_vals,e_vec = LA.eig(x.loc[i])
print(e_vals,e_vec)
except:
continue
If there are no NaN values present then you need not use the try and except instead go for only for loop.
edited Jan 21 at 5:09
answered Jan 20 at 5:48
SumanthSumanth
2169
2169
What I don't understand is why I can calculate a covariance matrix for each "window x 3" window but I cannot calculate a more general function. Of course, I can do another loop after that but it seems wasteful. Why go over the matrix twice?
– freevillage
Jan 21 at 0:46
add a comment |
What I don't understand is why I can calculate a covariance matrix for each "window x 3" window but I cannot calculate a more general function. Of course, I can do another loop after that but it seems wasteful. Why go over the matrix twice?
– freevillage
Jan 21 at 0:46
What I don't understand is why I can calculate a covariance matrix for each "window x 3" window but I cannot calculate a more general function. Of course, I can do another loop after that but it seems wasteful. Why go over the matrix twice?
– freevillage
Jan 21 at 0:46
What I don't understand is why I can calculate a covariance matrix for each "window x 3" window but I cannot calculate a more general function. Of course, I can do another loop after that but it seems wasteful. Why go over the matrix twice?
– freevillage
Jan 21 at 0:46
add a comment |
Thanks for contributing an answer to Stack Overflow!
- Please be sure to answer the question. Provide details and share your research!
But avoid …
- Asking for help, clarification, or responding to other answers.
- Making statements based on opinion; back them up with references or personal experience.
To learn more, see our tips on writing great answers.
Sign up or log in
StackExchange.ready(function () {
StackExchange.helpers.onClickDraftSave('#login-link');
});
Sign up using Google
Sign up using Facebook
Sign up using Email and Password
Post as a guest
Required, but never shown
StackExchange.ready(
function () {
StackExchange.openid.initPostLogin('.new-post-login', 'https%3a%2f%2fstackoverflow.com%2fquestions%2f54273340%2frolling-multidimensional-function-in-pandas%23new-answer', 'question_page');
}
);
Post as a guest
Required, but never shown
Sign up or log in
StackExchange.ready(function () {
StackExchange.helpers.onClickDraftSave('#login-link');
});
Sign up using Google
Sign up using Facebook
Sign up using Email and Password
Post as a guest
Required, but never shown
Sign up or log in
StackExchange.ready(function () {
StackExchange.helpers.onClickDraftSave('#login-link');
});
Sign up using Google
Sign up using Facebook
Sign up using Email and Password
Post as a guest
Required, but never shown
Sign up or log in
StackExchange.ready(function () {
StackExchange.helpers.onClickDraftSave('#login-link');
});
Sign up using Google
Sign up using Facebook
Sign up using Email and Password
Sign up using Google
Sign up using Facebook
Sign up using Email and Password
Post as a guest
Required, but never shown
Required, but never shown
Required, but never shown
Required, but never shown
Required, but never shown
Required, but never shown
Required, but never shown
Required, but never shown
Required, but never shown
FlEKOwU