Spark SQL filter multiple similar fields
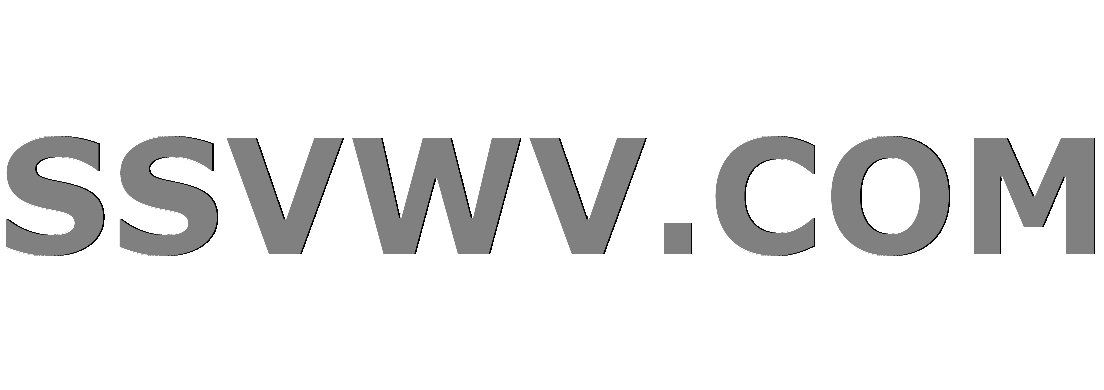
Multi tool use
Is there a better way to write a filter of multiple conditions which are similar in nature on a spark dataframe.
Assuming df is a spark dataframe having timestamp columns t1,t2,t3,t4.
val filteredDF=df.filter(col("t1").lt(current_date()-expr("INTERVAL 30 DAYS")) || col("t2").lt(current_date()-expr("INTERVAL 30 DAYS")) ||
col("t3").lt(current_date()-expr("INTERVAL 30 DAYS")) ||
col("t4").lt(current_date()-expr("INTERVAL 30 DAYS")))
Any better way to write the same. Since I'm new to scala, I kind of don't know the best practices to code in scala yet. Appreciate any help.
apache-spark apache-spark-sql
add a comment |
Is there a better way to write a filter of multiple conditions which are similar in nature on a spark dataframe.
Assuming df is a spark dataframe having timestamp columns t1,t2,t3,t4.
val filteredDF=df.filter(col("t1").lt(current_date()-expr("INTERVAL 30 DAYS")) || col("t2").lt(current_date()-expr("INTERVAL 30 DAYS")) ||
col("t3").lt(current_date()-expr("INTERVAL 30 DAYS")) ||
col("t4").lt(current_date()-expr("INTERVAL 30 DAYS")))
Any better way to write the same. Since I'm new to scala, I kind of don't know the best practices to code in scala yet. Appreciate any help.
apache-spark apache-spark-sql
add a comment |
Is there a better way to write a filter of multiple conditions which are similar in nature on a spark dataframe.
Assuming df is a spark dataframe having timestamp columns t1,t2,t3,t4.
val filteredDF=df.filter(col("t1").lt(current_date()-expr("INTERVAL 30 DAYS")) || col("t2").lt(current_date()-expr("INTERVAL 30 DAYS")) ||
col("t3").lt(current_date()-expr("INTERVAL 30 DAYS")) ||
col("t4").lt(current_date()-expr("INTERVAL 30 DAYS")))
Any better way to write the same. Since I'm new to scala, I kind of don't know the best practices to code in scala yet. Appreciate any help.
apache-spark apache-spark-sql
Is there a better way to write a filter of multiple conditions which are similar in nature on a spark dataframe.
Assuming df is a spark dataframe having timestamp columns t1,t2,t3,t4.
val filteredDF=df.filter(col("t1").lt(current_date()-expr("INTERVAL 30 DAYS")) || col("t2").lt(current_date()-expr("INTERVAL 30 DAYS")) ||
col("t3").lt(current_date()-expr("INTERVAL 30 DAYS")) ||
col("t4").lt(current_date()-expr("INTERVAL 30 DAYS")))
Any better way to write the same. Since I'm new to scala, I kind of don't know the best practices to code in scala yet. Appreciate any help.
apache-spark apache-spark-sql
apache-spark apache-spark-sql
asked Jan 19 at 6:24
Vikas JVikas J
891111
891111
add a comment |
add a comment |
2 Answers
2
active
oldest
votes
import df.sparkSession.implicits._
import org.apache.spark.sql.functions._
def filterDates(dates: Column*): Column =
dates
.map(_.lt(current_date()-expr("INTERVAL 30 DAYS")))
.reduce(_ or _)
val filteredDF = df.filter(filterDates($"t1", $"t2", $"t3", $"t4"))
I didn't even check if it compiles, but give or take a few typos it should do the job.
add a comment |
Check this out:
scala> val df =Seq( ( (Timestamp.valueOf("2019-01-01 01:02:03")), (Timestamp.valueOf("2019-01-10 01:02:03")), (Timestamp.valueOf("2019-01-15 01:02:03") ), (Timestamp.valueOf("2019-02-22 01:02:03")) ) ).toDF("t1","t2","t3","t4")
df: org.apache.spark.sql.DataFrame = [t1: timestamp, t2: timestamp ... 2 more fields]
scala> df.show(false)
+-------------------+-------------------+-------------------+-------------------+
|t1 |t2 |t3 |t4 |
+-------------------+-------------------+-------------------+-------------------+
|2019-01-01 01:02:03|2019-01-10 01:02:03|2019-01-15 01:02:03|2019-02-22 01:02:03|
+-------------------+-------------------+-------------------+-------------------+
scala> val ts_cols = df.dtypes.filter( _._2 == "TimestampType" ).map( _._1)
ts_cols: Array[String] = Array(t1, t2, t3, t4)
scala> val exp1 = ts_cols.map ( x=> col(x).lt(current_date()-expr("INTERVAL 30 DAYS")) ).reduce( _||_ )
exp1: org.apache.spark.sql.Column = ((((t1 < (current_date() - interval 4 weeks 2 days)) OR (t2 < (current_date() - interval 4 weeks 2 days))) OR (t3 < (current_date() - interval 4 weeks 2 days))) OR (t4 < (current_date() - interval 4 weeks 2 days)))
scala> df.select(col("*"),exp1.as("ts_comp") ).show(false)
+-------------------+-------------------+-------------------+-------------------+-------+
|t1 |t2 |t3 |t4 |ts_comp|
+-------------------+-------------------+-------------------+-------------------+-------+
|2019-01-01 01:02:03|2019-01-10 01:02:03|2019-01-15 01:02:03|2019-02-22 01:02:03|false |
+-------------------+-------------------+-------------------+-------------------+-------+
true
test case
scala> val df2 =Seq( ( (Timestamp.valueOf("2018-01-01 01:02:03")), (Timestamp.valueOf("2018-01-10 01:02:03")), (Timestamp.valueOf("2018-01-15 01:
02:03") ), (Timestamp.valueOf("2018-02-22 01:02:03")) ) ).toDF("t1","t2","t3","t4")
df2: org.apache.spark.sql.DataFrame = [t1: timestamp, t2: timestamp ... 2 more fields]
scala> df2.select(col("*"),exp1.as("ts_comp") ).show(false)
+-------------------+-------------------+-------------------+-------------------+-------+
|t1 |t2 |t3 |t4 |ts_comp|
+-------------------+-------------------+-------------------+-------------------+-------+
|2018-01-01 01:02:03|2018-01-10 01:02:03|2018-01-15 01:02:03|2018-02-22 01:02:03|true |
+-------------------+-------------------+-------------------+-------------------+-------+
scala>
add a comment |
Your Answer
StackExchange.ifUsing("editor", function () {
StackExchange.using("externalEditor", function () {
StackExchange.using("snippets", function () {
StackExchange.snippets.init();
});
});
}, "code-snippets");
StackExchange.ready(function() {
var channelOptions = {
tags: "".split(" "),
id: "1"
};
initTagRenderer("".split(" "), "".split(" "), channelOptions);
StackExchange.using("externalEditor", function() {
// Have to fire editor after snippets, if snippets enabled
if (StackExchange.settings.snippets.snippetsEnabled) {
StackExchange.using("snippets", function() {
createEditor();
});
}
else {
createEditor();
}
});
function createEditor() {
StackExchange.prepareEditor({
heartbeatType: 'answer',
autoActivateHeartbeat: false,
convertImagesToLinks: true,
noModals: true,
showLowRepImageUploadWarning: true,
reputationToPostImages: 10,
bindNavPrevention: true,
postfix: "",
imageUploader: {
brandingHtml: "Powered by u003ca class="icon-imgur-white" href="https://imgur.com/"u003eu003c/au003e",
contentPolicyHtml: "User contributions licensed under u003ca href="https://creativecommons.org/licenses/by-sa/3.0/"u003ecc by-sa 3.0 with attribution requiredu003c/au003e u003ca href="https://stackoverflow.com/legal/content-policy"u003e(content policy)u003c/au003e",
allowUrls: true
},
onDemand: true,
discardSelector: ".discard-answer"
,immediatelyShowMarkdownHelp:true
});
}
});
Sign up or log in
StackExchange.ready(function () {
StackExchange.helpers.onClickDraftSave('#login-link');
});
Sign up using Google
Sign up using Facebook
Sign up using Email and Password
Post as a guest
Required, but never shown
StackExchange.ready(
function () {
StackExchange.openid.initPostLogin('.new-post-login', 'https%3a%2f%2fstackoverflow.com%2fquestions%2f54264624%2fspark-sql-filter-multiple-similar-fields%23new-answer', 'question_page');
}
);
Post as a guest
Required, but never shown
2 Answers
2
active
oldest
votes
2 Answers
2
active
oldest
votes
active
oldest
votes
active
oldest
votes
import df.sparkSession.implicits._
import org.apache.spark.sql.functions._
def filterDates(dates: Column*): Column =
dates
.map(_.lt(current_date()-expr("INTERVAL 30 DAYS")))
.reduce(_ or _)
val filteredDF = df.filter(filterDates($"t1", $"t2", $"t3", $"t4"))
I didn't even check if it compiles, but give or take a few typos it should do the job.
add a comment |
import df.sparkSession.implicits._
import org.apache.spark.sql.functions._
def filterDates(dates: Column*): Column =
dates
.map(_.lt(current_date()-expr("INTERVAL 30 DAYS")))
.reduce(_ or _)
val filteredDF = df.filter(filterDates($"t1", $"t2", $"t3", $"t4"))
I didn't even check if it compiles, but give or take a few typos it should do the job.
add a comment |
import df.sparkSession.implicits._
import org.apache.spark.sql.functions._
def filterDates(dates: Column*): Column =
dates
.map(_.lt(current_date()-expr("INTERVAL 30 DAYS")))
.reduce(_ or _)
val filteredDF = df.filter(filterDates($"t1", $"t2", $"t3", $"t4"))
I didn't even check if it compiles, but give or take a few typos it should do the job.
import df.sparkSession.implicits._
import org.apache.spark.sql.functions._
def filterDates(dates: Column*): Column =
dates
.map(_.lt(current_date()-expr("INTERVAL 30 DAYS")))
.reduce(_ or _)
val filteredDF = df.filter(filterDates($"t1", $"t2", $"t3", $"t4"))
I didn't even check if it compiles, but give or take a few typos it should do the job.
answered Jan 19 at 7:29


shay__shay__
2,133622
2,133622
add a comment |
add a comment |
Check this out:
scala> val df =Seq( ( (Timestamp.valueOf("2019-01-01 01:02:03")), (Timestamp.valueOf("2019-01-10 01:02:03")), (Timestamp.valueOf("2019-01-15 01:02:03") ), (Timestamp.valueOf("2019-02-22 01:02:03")) ) ).toDF("t1","t2","t3","t4")
df: org.apache.spark.sql.DataFrame = [t1: timestamp, t2: timestamp ... 2 more fields]
scala> df.show(false)
+-------------------+-------------------+-------------------+-------------------+
|t1 |t2 |t3 |t4 |
+-------------------+-------------------+-------------------+-------------------+
|2019-01-01 01:02:03|2019-01-10 01:02:03|2019-01-15 01:02:03|2019-02-22 01:02:03|
+-------------------+-------------------+-------------------+-------------------+
scala> val ts_cols = df.dtypes.filter( _._2 == "TimestampType" ).map( _._1)
ts_cols: Array[String] = Array(t1, t2, t3, t4)
scala> val exp1 = ts_cols.map ( x=> col(x).lt(current_date()-expr("INTERVAL 30 DAYS")) ).reduce( _||_ )
exp1: org.apache.spark.sql.Column = ((((t1 < (current_date() - interval 4 weeks 2 days)) OR (t2 < (current_date() - interval 4 weeks 2 days))) OR (t3 < (current_date() - interval 4 weeks 2 days))) OR (t4 < (current_date() - interval 4 weeks 2 days)))
scala> df.select(col("*"),exp1.as("ts_comp") ).show(false)
+-------------------+-------------------+-------------------+-------------------+-------+
|t1 |t2 |t3 |t4 |ts_comp|
+-------------------+-------------------+-------------------+-------------------+-------+
|2019-01-01 01:02:03|2019-01-10 01:02:03|2019-01-15 01:02:03|2019-02-22 01:02:03|false |
+-------------------+-------------------+-------------------+-------------------+-------+
true
test case
scala> val df2 =Seq( ( (Timestamp.valueOf("2018-01-01 01:02:03")), (Timestamp.valueOf("2018-01-10 01:02:03")), (Timestamp.valueOf("2018-01-15 01:
02:03") ), (Timestamp.valueOf("2018-02-22 01:02:03")) ) ).toDF("t1","t2","t3","t4")
df2: org.apache.spark.sql.DataFrame = [t1: timestamp, t2: timestamp ... 2 more fields]
scala> df2.select(col("*"),exp1.as("ts_comp") ).show(false)
+-------------------+-------------------+-------------------+-------------------+-------+
|t1 |t2 |t3 |t4 |ts_comp|
+-------------------+-------------------+-------------------+-------------------+-------+
|2018-01-01 01:02:03|2018-01-10 01:02:03|2018-01-15 01:02:03|2018-02-22 01:02:03|true |
+-------------------+-------------------+-------------------+-------------------+-------+
scala>
add a comment |
Check this out:
scala> val df =Seq( ( (Timestamp.valueOf("2019-01-01 01:02:03")), (Timestamp.valueOf("2019-01-10 01:02:03")), (Timestamp.valueOf("2019-01-15 01:02:03") ), (Timestamp.valueOf("2019-02-22 01:02:03")) ) ).toDF("t1","t2","t3","t4")
df: org.apache.spark.sql.DataFrame = [t1: timestamp, t2: timestamp ... 2 more fields]
scala> df.show(false)
+-------------------+-------------------+-------------------+-------------------+
|t1 |t2 |t3 |t4 |
+-------------------+-------------------+-------------------+-------------------+
|2019-01-01 01:02:03|2019-01-10 01:02:03|2019-01-15 01:02:03|2019-02-22 01:02:03|
+-------------------+-------------------+-------------------+-------------------+
scala> val ts_cols = df.dtypes.filter( _._2 == "TimestampType" ).map( _._1)
ts_cols: Array[String] = Array(t1, t2, t3, t4)
scala> val exp1 = ts_cols.map ( x=> col(x).lt(current_date()-expr("INTERVAL 30 DAYS")) ).reduce( _||_ )
exp1: org.apache.spark.sql.Column = ((((t1 < (current_date() - interval 4 weeks 2 days)) OR (t2 < (current_date() - interval 4 weeks 2 days))) OR (t3 < (current_date() - interval 4 weeks 2 days))) OR (t4 < (current_date() - interval 4 weeks 2 days)))
scala> df.select(col("*"),exp1.as("ts_comp") ).show(false)
+-------------------+-------------------+-------------------+-------------------+-------+
|t1 |t2 |t3 |t4 |ts_comp|
+-------------------+-------------------+-------------------+-------------------+-------+
|2019-01-01 01:02:03|2019-01-10 01:02:03|2019-01-15 01:02:03|2019-02-22 01:02:03|false |
+-------------------+-------------------+-------------------+-------------------+-------+
true
test case
scala> val df2 =Seq( ( (Timestamp.valueOf("2018-01-01 01:02:03")), (Timestamp.valueOf("2018-01-10 01:02:03")), (Timestamp.valueOf("2018-01-15 01:
02:03") ), (Timestamp.valueOf("2018-02-22 01:02:03")) ) ).toDF("t1","t2","t3","t4")
df2: org.apache.spark.sql.DataFrame = [t1: timestamp, t2: timestamp ... 2 more fields]
scala> df2.select(col("*"),exp1.as("ts_comp") ).show(false)
+-------------------+-------------------+-------------------+-------------------+-------+
|t1 |t2 |t3 |t4 |ts_comp|
+-------------------+-------------------+-------------------+-------------------+-------+
|2018-01-01 01:02:03|2018-01-10 01:02:03|2018-01-15 01:02:03|2018-02-22 01:02:03|true |
+-------------------+-------------------+-------------------+-------------------+-------+
scala>
add a comment |
Check this out:
scala> val df =Seq( ( (Timestamp.valueOf("2019-01-01 01:02:03")), (Timestamp.valueOf("2019-01-10 01:02:03")), (Timestamp.valueOf("2019-01-15 01:02:03") ), (Timestamp.valueOf("2019-02-22 01:02:03")) ) ).toDF("t1","t2","t3","t4")
df: org.apache.spark.sql.DataFrame = [t1: timestamp, t2: timestamp ... 2 more fields]
scala> df.show(false)
+-------------------+-------------------+-------------------+-------------------+
|t1 |t2 |t3 |t4 |
+-------------------+-------------------+-------------------+-------------------+
|2019-01-01 01:02:03|2019-01-10 01:02:03|2019-01-15 01:02:03|2019-02-22 01:02:03|
+-------------------+-------------------+-------------------+-------------------+
scala> val ts_cols = df.dtypes.filter( _._2 == "TimestampType" ).map( _._1)
ts_cols: Array[String] = Array(t1, t2, t3, t4)
scala> val exp1 = ts_cols.map ( x=> col(x).lt(current_date()-expr("INTERVAL 30 DAYS")) ).reduce( _||_ )
exp1: org.apache.spark.sql.Column = ((((t1 < (current_date() - interval 4 weeks 2 days)) OR (t2 < (current_date() - interval 4 weeks 2 days))) OR (t3 < (current_date() - interval 4 weeks 2 days))) OR (t4 < (current_date() - interval 4 weeks 2 days)))
scala> df.select(col("*"),exp1.as("ts_comp") ).show(false)
+-------------------+-------------------+-------------------+-------------------+-------+
|t1 |t2 |t3 |t4 |ts_comp|
+-------------------+-------------------+-------------------+-------------------+-------+
|2019-01-01 01:02:03|2019-01-10 01:02:03|2019-01-15 01:02:03|2019-02-22 01:02:03|false |
+-------------------+-------------------+-------------------+-------------------+-------+
true
test case
scala> val df2 =Seq( ( (Timestamp.valueOf("2018-01-01 01:02:03")), (Timestamp.valueOf("2018-01-10 01:02:03")), (Timestamp.valueOf("2018-01-15 01:
02:03") ), (Timestamp.valueOf("2018-02-22 01:02:03")) ) ).toDF("t1","t2","t3","t4")
df2: org.apache.spark.sql.DataFrame = [t1: timestamp, t2: timestamp ... 2 more fields]
scala> df2.select(col("*"),exp1.as("ts_comp") ).show(false)
+-------------------+-------------------+-------------------+-------------------+-------+
|t1 |t2 |t3 |t4 |ts_comp|
+-------------------+-------------------+-------------------+-------------------+-------+
|2018-01-01 01:02:03|2018-01-10 01:02:03|2018-01-15 01:02:03|2018-02-22 01:02:03|true |
+-------------------+-------------------+-------------------+-------------------+-------+
scala>
Check this out:
scala> val df =Seq( ( (Timestamp.valueOf("2019-01-01 01:02:03")), (Timestamp.valueOf("2019-01-10 01:02:03")), (Timestamp.valueOf("2019-01-15 01:02:03") ), (Timestamp.valueOf("2019-02-22 01:02:03")) ) ).toDF("t1","t2","t3","t4")
df: org.apache.spark.sql.DataFrame = [t1: timestamp, t2: timestamp ... 2 more fields]
scala> df.show(false)
+-------------------+-------------------+-------------------+-------------------+
|t1 |t2 |t3 |t4 |
+-------------------+-------------------+-------------------+-------------------+
|2019-01-01 01:02:03|2019-01-10 01:02:03|2019-01-15 01:02:03|2019-02-22 01:02:03|
+-------------------+-------------------+-------------------+-------------------+
scala> val ts_cols = df.dtypes.filter( _._2 == "TimestampType" ).map( _._1)
ts_cols: Array[String] = Array(t1, t2, t3, t4)
scala> val exp1 = ts_cols.map ( x=> col(x).lt(current_date()-expr("INTERVAL 30 DAYS")) ).reduce( _||_ )
exp1: org.apache.spark.sql.Column = ((((t1 < (current_date() - interval 4 weeks 2 days)) OR (t2 < (current_date() - interval 4 weeks 2 days))) OR (t3 < (current_date() - interval 4 weeks 2 days))) OR (t4 < (current_date() - interval 4 weeks 2 days)))
scala> df.select(col("*"),exp1.as("ts_comp") ).show(false)
+-------------------+-------------------+-------------------+-------------------+-------+
|t1 |t2 |t3 |t4 |ts_comp|
+-------------------+-------------------+-------------------+-------------------+-------+
|2019-01-01 01:02:03|2019-01-10 01:02:03|2019-01-15 01:02:03|2019-02-22 01:02:03|false |
+-------------------+-------------------+-------------------+-------------------+-------+
true
test case
scala> val df2 =Seq( ( (Timestamp.valueOf("2018-01-01 01:02:03")), (Timestamp.valueOf("2018-01-10 01:02:03")), (Timestamp.valueOf("2018-01-15 01:
02:03") ), (Timestamp.valueOf("2018-02-22 01:02:03")) ) ).toDF("t1","t2","t3","t4")
df2: org.apache.spark.sql.DataFrame = [t1: timestamp, t2: timestamp ... 2 more fields]
scala> df2.select(col("*"),exp1.as("ts_comp") ).show(false)
+-------------------+-------------------+-------------------+-------------------+-------+
|t1 |t2 |t3 |t4 |ts_comp|
+-------------------+-------------------+-------------------+-------------------+-------+
|2018-01-01 01:02:03|2018-01-10 01:02:03|2018-01-15 01:02:03|2018-02-22 01:02:03|true |
+-------------------+-------------------+-------------------+-------------------+-------+
scala>
answered Jan 19 at 13:45
stack0114106stack0114106
3,1142417
3,1142417
add a comment |
add a comment |
Thanks for contributing an answer to Stack Overflow!
- Please be sure to answer the question. Provide details and share your research!
But avoid …
- Asking for help, clarification, or responding to other answers.
- Making statements based on opinion; back them up with references or personal experience.
To learn more, see our tips on writing great answers.
Sign up or log in
StackExchange.ready(function () {
StackExchange.helpers.onClickDraftSave('#login-link');
});
Sign up using Google
Sign up using Facebook
Sign up using Email and Password
Post as a guest
Required, but never shown
StackExchange.ready(
function () {
StackExchange.openid.initPostLogin('.new-post-login', 'https%3a%2f%2fstackoverflow.com%2fquestions%2f54264624%2fspark-sql-filter-multiple-similar-fields%23new-answer', 'question_page');
}
);
Post as a guest
Required, but never shown
Sign up or log in
StackExchange.ready(function () {
StackExchange.helpers.onClickDraftSave('#login-link');
});
Sign up using Google
Sign up using Facebook
Sign up using Email and Password
Post as a guest
Required, but never shown
Sign up or log in
StackExchange.ready(function () {
StackExchange.helpers.onClickDraftSave('#login-link');
});
Sign up using Google
Sign up using Facebook
Sign up using Email and Password
Post as a guest
Required, but never shown
Sign up or log in
StackExchange.ready(function () {
StackExchange.helpers.onClickDraftSave('#login-link');
});
Sign up using Google
Sign up using Facebook
Sign up using Email and Password
Sign up using Google
Sign up using Facebook
Sign up using Email and Password
Post as a guest
Required, but never shown
Required, but never shown
Required, but never shown
Required, but never shown
Required, but never shown
Required, but never shown
Required, but never shown
Required, but never shown
Required, but never shown
GhL2ZXc,lIdZCt1i4Bm,W,omoK7zxFopRJD